論文
2019
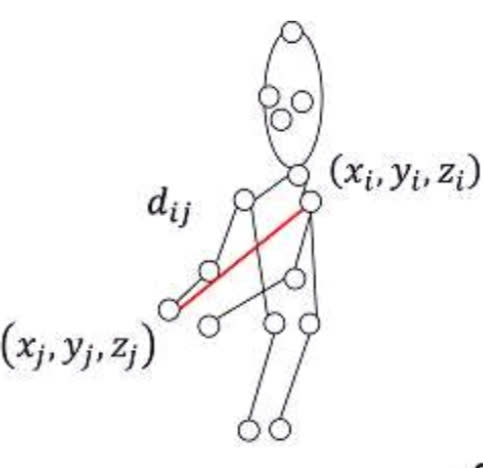
Temporal Distance Matrices for Squat Classification
Ryoji Ogata, Edgar Simo-Serra, Satoshi Iizuka, Hiroshi Ishikawa
Conference in Computer Vision and Pattern Recognition Workshops (CVPRW), 2019
@InProceedings{OgataCVPRW2019, author = {Ryoji Ogata and Edgar Simo-Serra and Satoshi Iizuka and Hiroshi Ishikawa}, title = {Temporal Distance Matrices for Squat Classification}, booktitle = "Proceedings of the Conference on Computer Vision and Pattern Recognition Workshops (CVPRW)", year = 2019, }
When working out, it is necessary to perform the same action many times for it to have effect. If the action, such as squats or bench pressing, is performed with poor form, it can lead to serious injuries in the long term. With the prevention of such harm in mind, we present an action dataset of videos where different types of poor form are annotated for a diversity of subjects and backgrounds, and propose a model for the form-classification task based on temporal distance matrices, both in the case of squats. We first run a 3D pose detector, then normalize the pose and compute the distance matrix, in which each element represents the normalized distance between two joints. This representation is invariant under global translation and rotation, as well as robust to individual differences, allowing for better generalization to real world data. Our classification model consists of a CNN with 1D convolutions. Results show that our method significantly outperforms existing approaches for the task.